06.01 - CLUSTERING
Contents
06.01 - CLUSTERING¶
!wget --no-cache -O init.py -q https://raw.githubusercontent.com/fagonzalezo/ai4eng-unal/main/content/init.py
import init; init.init(force_download=False); init.get_weblink()
import warnings
warnings.filterwarnings('ignore')
from local.lib import mlutils
import numpy as np
import pandas as pd
import matplotlib.pyplot as plt
from sklearn.metrics import silhouette_samples, silhouette_score
from sklearn.datasets import make_blobs, make_moons
from sklearn.cluster import KMeans, SpectralClustering, AgglomerativeClustering, DBSCAN
from IPython.display import HTML, Image
%matplotlib inline
Referencias generales¶
Image(filename='local/imgs/learning.jpg', width=600)
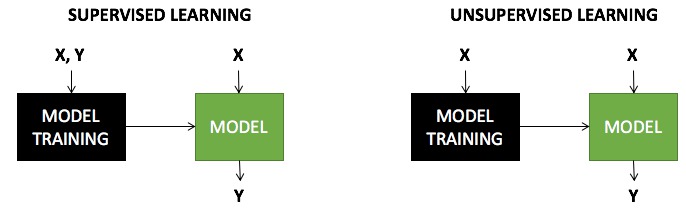
Qué es clustering?
Objetivo: agrupar objetos físicos o obstractos en clases de objetos similares
Es una tarea NO SUPERVISADA \(\rightarrow\) no sabemos a priorí cómo clasificar nuestros objetos
Es una tarea NO COMPLETAMENTE DEFINIDA \(\rightarrow\) ¿Cómo cuantificamos el desempeño de un resultado de clustering?
¿Qué definición de similitud establecemos?
Ejemplos de aplicaciones de clustering
Taxonomías en biología, agrupaciones por similitud biológica, o incluso genética (big data!!)
Páginas similares para estructurar resultados de búsquedas (p.ej. La búsqueda de “película” podría devolver resultados agrupados por descripciones similares.
Segmentación de clientes o usuarios por un criterio de similitud definido.
Intuición¶
¿Qué grupos harías con los siguientes datos?, ¿Cómo sería el proceso?
X = pd.read_csv("local/data/cluster1.csv").values+15
plt.scatter(X[:,0], X[:,1])
plt.xlabel("anchura del trilobite")
plt.ylabel("largo del trilobite");
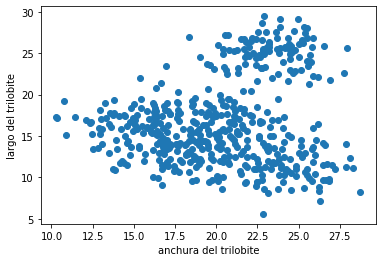
pseudo código
input:
X: datos
k: número de clusters deseados
algoritmo:
1. selecciona k centroides aleatoriamente
2. repite hasta que los k centroides no cambien:
3. establece k clusters asignado cada dato al centroide más cercano
4. recalcula el centroide de cada cluster como el promedio de los datos
h = '<iframe width="560" height="315" src="https://www.youtube.com/embed/BVFG7fd1H30" frameborder="0" allow="autoplay; encrypted-media" allowfullscreen></iframe>'
HTML(h)
Métodos basados en centroides - KMeans¶
X = pd.read_csv("local/data/cluster1.csv").values+15
X.shape
(500, 2)
X[:10]
array([[16.06479619, 15.64135085],
[23.136145 , 13.27029397],
[21.2907726 , 25.33499533],
[24.4739302 , 24.28665806],
[16.29365483, 17.15224079],
[24.3799962 , 21.6928672 ],
[22.96399174, 13.7776461 ],
[14.59797792, 16.23800883],
[21.68532999, 18.31450552],
[21.74355998, 23.70715595]])
n_clusters = 2
km = KMeans(n_clusters=n_clusters)
km.fit(X)
y = km.predict(X)
y.shape
(500,)
pd.Series(y).value_counts()
0 392
1 108
dtype: int64
km.cluster_centers_
array([[19.5061742 , 14.31526768],
[23.01615594, 24.85357474]])
def plot_clusters(X,y):
n_clusters = len(np.unique(y))
cmap = plt.cm.bwr if n_clusters==2 else plt.cm.plasma
cmap((y*255./(n_clusters-1)).astype(int))
for i in np.unique(y):
col = cmap((i*255./(n_clusters-1)).astype(int))
Xr = X[y==i]
plt.scatter(Xr[:,0], Xr[:,1], color=col, label="cluster %d"%i, alpha=.5)
plt.scatter(km.cluster_centers_[:,0], km.cluster_centers_[:,1],marker="x", lw=5, s=200, color="black")
plt.legend()
plt.xlabel("anchura del trilobite")
plt.ylabel("largo del trilobite");
plot_clusters(X,y)
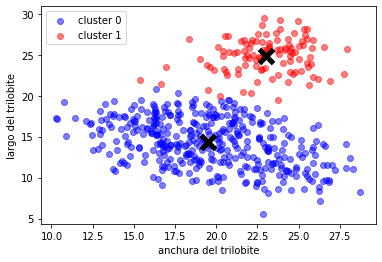
observa cómo KMeans agrupa datos en 2D con diferentes números de clusters
X = pd.read_csv("local/data/cluster1.csv").values
mlutils.experiment_number_of_clusters(X, KMeans(), show_metric=False)
100% (8 of 8) |##########################| Elapsed Time: 0:00:00 Time: 0:00:00
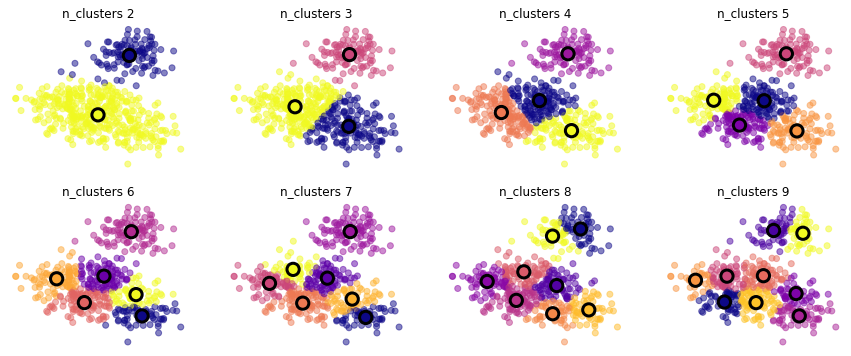
exerimenta con distintos datasets sintéticos¶
cambia
cluster_std
ycenters
enmake_blobs
para generar datasets con distintas distribucionescuál es el númer de clusters natural que usarías? por qué es natural?
X,_ = make_blobs(500, cluster_std=1, centers=7)
plt.scatter(X[:,0], X[:,1], color="black", alpha=.5)
<matplotlib.collections.PathCollection at 0x7f5550823490>
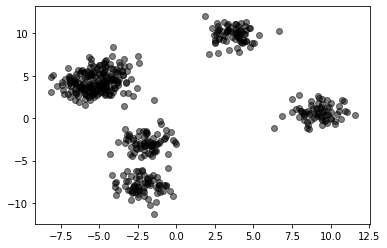
mlutils.experiment_number_of_clusters(X, KMeans(), show_metric=False)
100% (8 of 8) |##########################| Elapsed Time: 0:00:00 Time: 0:00:00
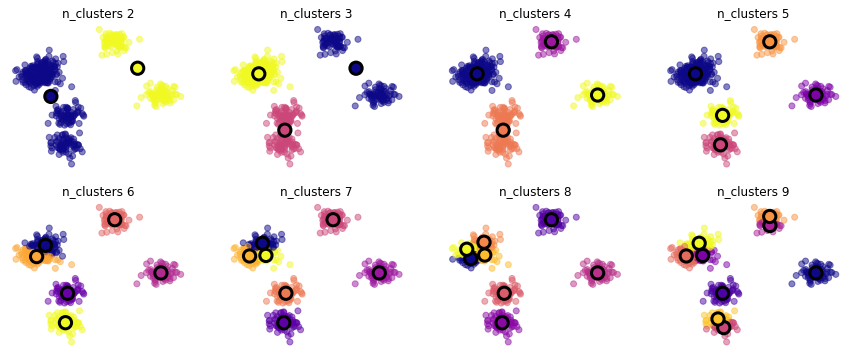
Cómo seleccionar el número de clusters? Consulta Silhouette Coefficient¶
X = pd.read_csv("local/data/cluster1.csv").values
plt.scatter(X[:,0], X[:,1], alpha=.5, color="black")
<matplotlib.collections.PathCollection at 0x7f554ee91d10>
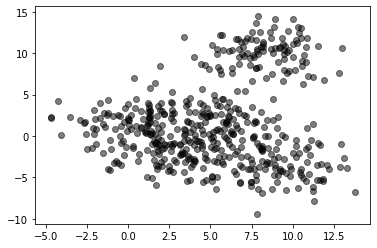
def silhouette_analysis(X):
range_n_clusters = [2, 3, 4, 5, 6]
for n_clusters in range_n_clusters:
# Create a subplot with 1 row and 2 columns
fig, (ax1, ax2) = plt.subplots(1, 2)
fig.set_size_inches(19, 4)
# The 1st subplot is the silhouette plot
# The silhouette coefficient can range from -1, 1 but in this example all
# lie within [-0.1, 1]
ax1.set_xlim([-0.1, 1])
# The (n_clusters+1)*10 is for inserting blank space between silhouette
# plots of individual clusters, to demarcate them clearly.
ax1.set_ylim([0, len(X) + (n_clusters + 1) * 10])
# Initialize the clusterer with n_clusters value and a random generator
# seed of 10 for reproducibility.
clusterer = KMeans(n_clusters=n_clusters, random_state=10)
cluster_labels = clusterer.fit_predict(X)
# The silhouette_score gives the average value for all the samples.
# This gives a perspective into the density and separation of the formed
# clusters
silhouette_avg = silhouette_score(X, cluster_labels)
# Compute the silhouette scores for each sample
sample_silhouette_values = silhouette_samples(X, cluster_labels)
y_lower = 10
for i in range(n_clusters):
# Aggregate the silhouette scores for samples belonging to
# cluster i, and sort them
ith_cluster_silhouette_values = \
sample_silhouette_values[cluster_labels == i]
ith_cluster_silhouette_values.sort()
size_cluster_i = ith_cluster_silhouette_values.shape[0]
y_upper = y_lower + size_cluster_i
color = plt.cm.nipy_spectral(float(i) / n_clusters)
ax1.fill_betweenx(np.arange(y_lower, y_upper),
0, ith_cluster_silhouette_values,
facecolor=color, edgecolor=color, alpha=0.7)
# Label the silhouette plots with their cluster numbers at the middle
ax1.text(-0.05, y_lower + 0.5 * size_cluster_i, str(i))
# Compute the new y_lower for next plot
y_lower = y_upper + 10 # 10 for the 0 samples
ax1.set_title("The silhouette plot for the various clusters.")
ax1.set_xlabel("The silhouette coefficient values")
ax1.set_ylabel("Cluster label")
# The vertical line for average silhouette score of all the values
ax1.axvline(x=silhouette_avg, color="red", linestyle="--")
ax1.set_yticks([]) # Clear the yaxis labels / ticks
ax1.set_xticks([-0.1, 0, 0.2, 0.4, 0.6, 0.8, 1])
# 2nd Plot showing the actual clusters formed
colors = plt.cm.nipy_spectral(cluster_labels.astype(float) / n_clusters)
ax2.scatter(X[:, 0], X[:, 1], marker='.', s=30, lw=0, alpha=0.7,
c=colors, edgecolor='k')
# Labeling the clusters
centers = clusterer.cluster_centers_
# Draw white circles at cluster centers
ax2.scatter(centers[:, 0], centers[:, 1], marker='o',
c="white", alpha=1, s=200, edgecolor='k')
for i, c in enumerate(centers):
ax2.scatter(c[0], c[1], marker='$%d$' % i, alpha=1,
s=50, edgecolor='k')
ax2.set_title("The visualization of the clustered data.")
ax2.set_xlabel("Feature space for the 1st feature")
ax2.set_ylabel("Feature space for the 2nd feature")
plt.suptitle(("Silhouette analysis for %s clustering on sample data "
"with n_clusters = %d, score = %.3f" % (clusterer.__class__.__name__, n_clusters,silhouette_avg)),
fontsize=14, fontweight='bold')
silhouette_analysis(X)
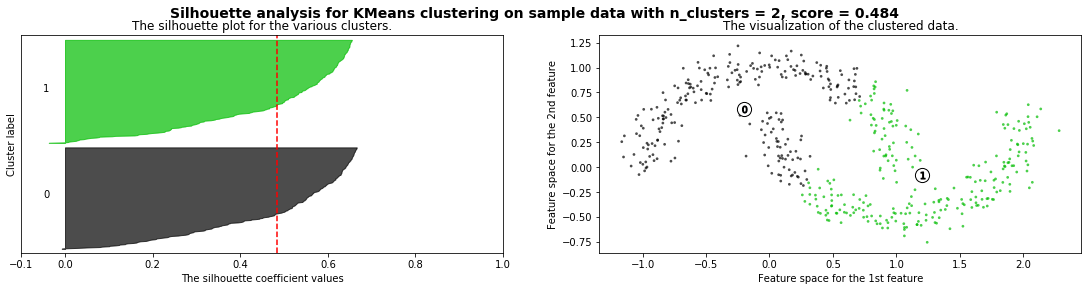
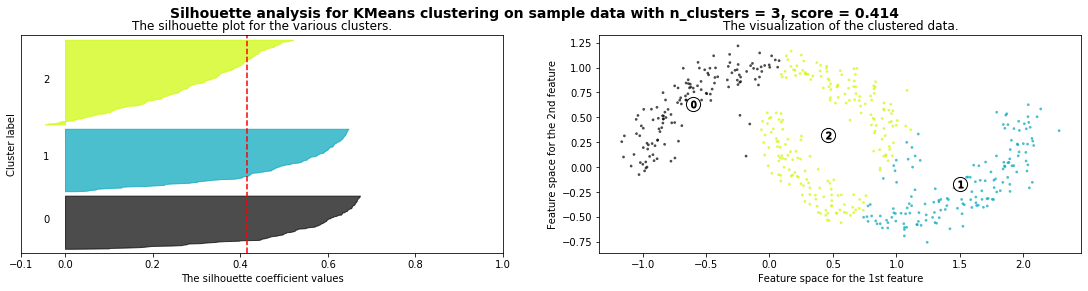
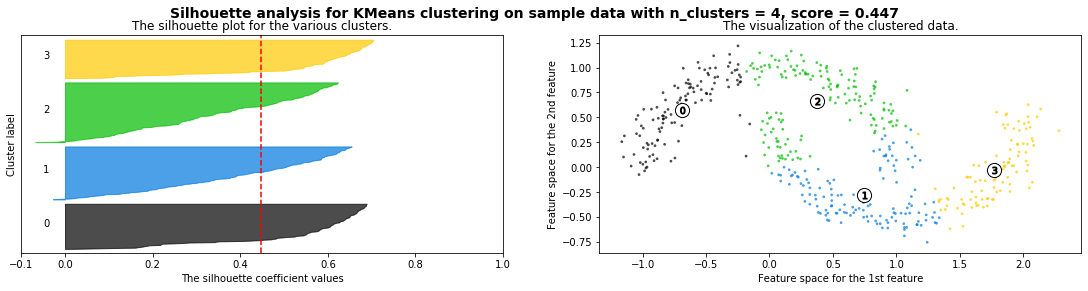
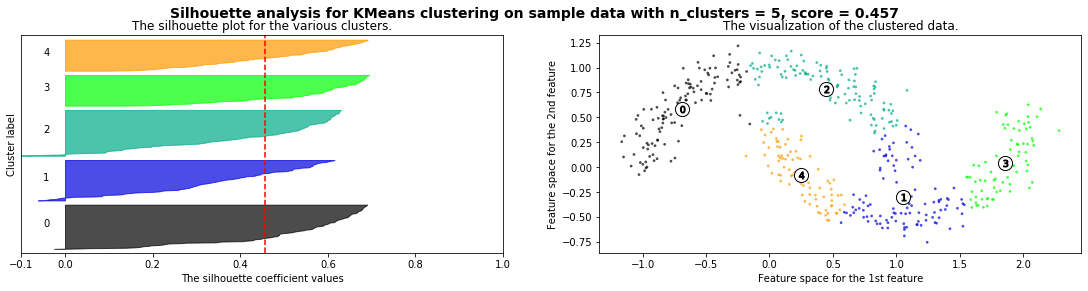
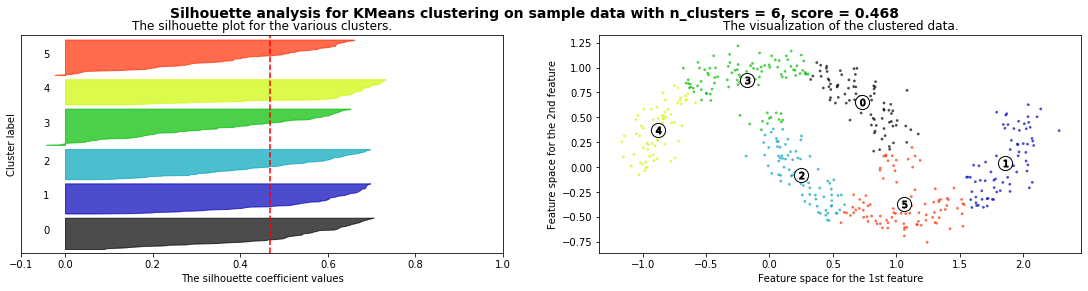
X,_ = make_blobs(500, cluster_std=.8, centers=4)
plt.scatter(X[:,0], X[:,1], alpha=.5, color="black")
<matplotlib.collections.PathCollection at 0x7f93d8486f90>
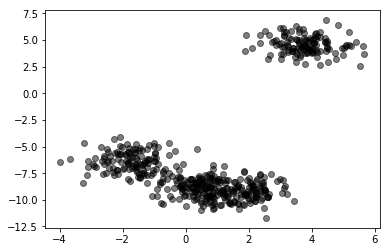
silhouette_analysis(X)
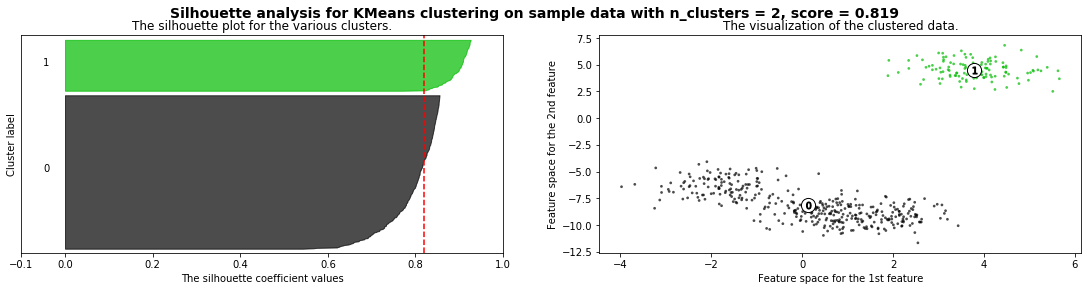
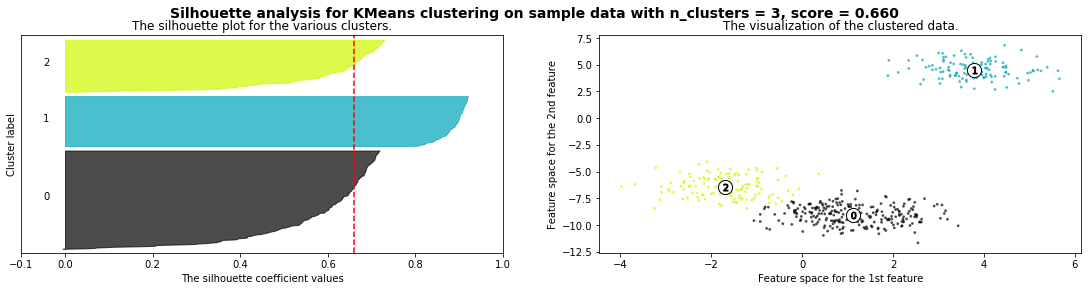
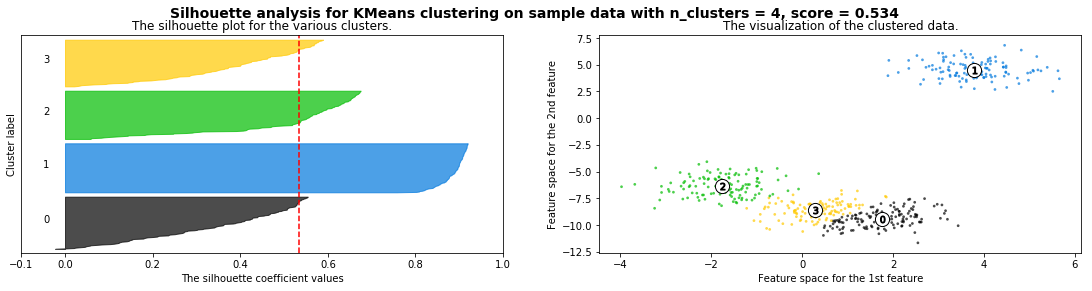
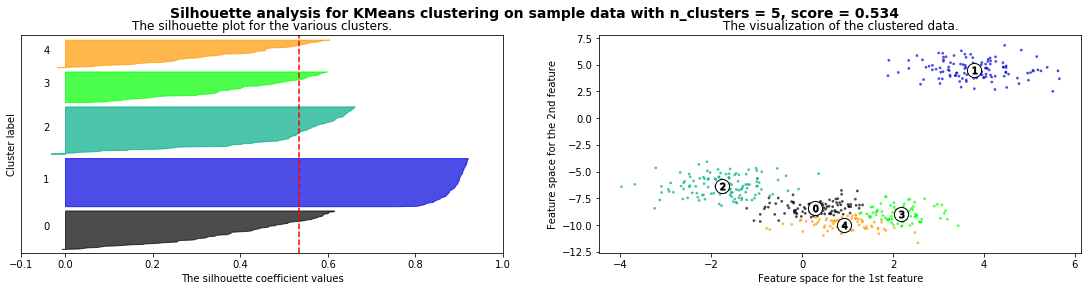
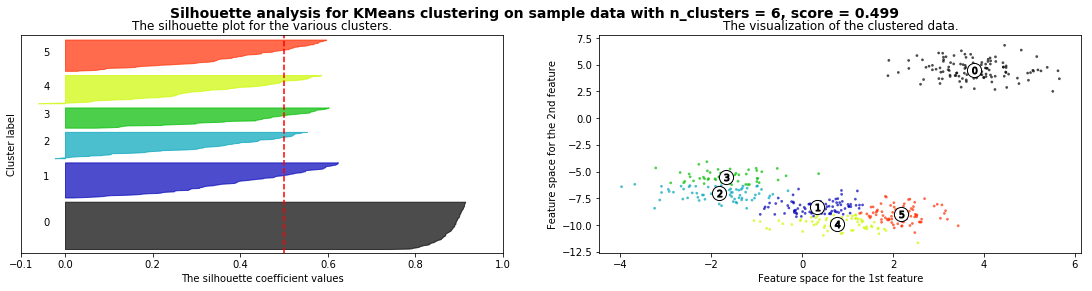
X,_ = make_moons(500, noise=.1)
plt.scatter(X[:,0], X[:,1], color="black", alpha=.5)
<matplotlib.collections.PathCollection at 0x7f554ee015d0>
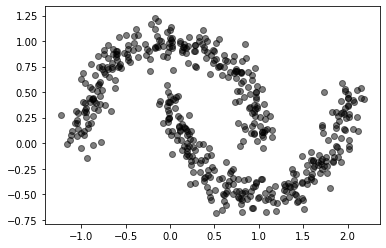
son naturales los clusters formados con los siguientes datos?
X,_ = make_moons(500, noise=.1)
mlutils.plot_cluster_predictions(KMeans(), X, n_clusters=2,cmap=plt.cm.bwr, show_metric=True)
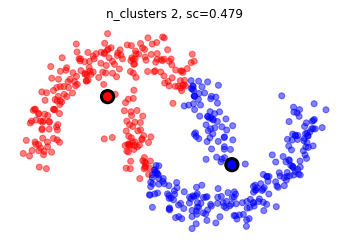
mlutils.plot_cluster_predictions(KMeans(), X, n_clusters=4,cmap=plt.cm.bwr, show_metric=True)
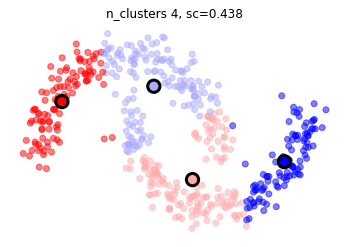
Métodos basados en densidad - DBSCAN¶
es necesario especificar \(\epsilon\) (radio máximo de una vecindad) y min_samples
.
cómo se comporta la métrica de silueta? qué métrica usarías?
X,_ = make_moons(500, noise=.1)
dbs = DBSCAN(eps=.15, min_samples=4, metric='euclidean')
mlutils.plot_cluster_predictions(dbs, X, cmap=plt.cm.plasma, show_metric=True)
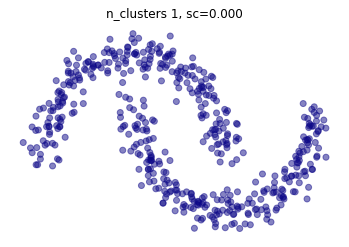
muy sensible a \(\epsilon\). observa también que la métrica de silueta es muy sensible a los outliers.
plt.figure(figsize=(15,3))
for i,eps in enumerate([.1,.15,.2]):
plt.subplot(1,3,i+1)
mlutils.plot_cluster_predictions(DBSCAN(eps=eps, min_samples=4, metric='euclidean'), X,
cmap=plt.cm.plasma, show_metric=True, title_str=", eps=%.2f"%eps)
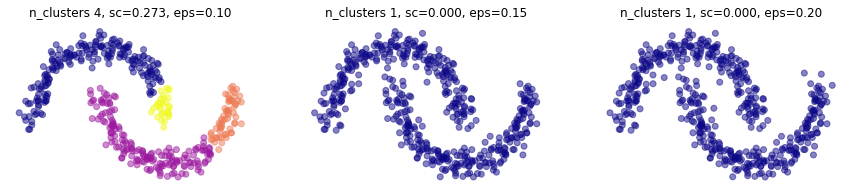
plt.figure(figsize=(15,3))
for i,min_samples in enumerate([1,4,10]):
plt.subplot(1,3,i+1)
mlutils.plot_cluster_predictions(DBSCAN(eps=.15, min_samples=min_samples, metric='euclidean'), X,
cmap=plt.cm.plasma, show_metric=True, title_str=", eps=%.2f"%eps)
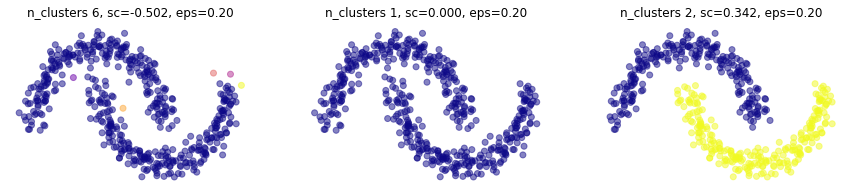
Métodos basados en conectividad - Agglomerative Clustering (Hierarchical)¶
consulta los parámetros en sklearn doc para describir una caracterización de la estructura subyacente.
observa cómo podemos construir una matrix de conectividad de k-vecinos alrededor de cualquier punto.
X,_ = make_moons(500, noise=.1)
from sklearn.neighbors import kneighbors_graph
i = np.random.randint(len(X))
knn_graph = kneighbors_graph(X, 50, include_self=False)
nn = X[knn_graph[i].toarray()[0].astype(bool)]
plt.scatter(nn[:,0], nn[:,1], color="blue", alpha=1)
plt.scatter(X[:,0], X[:,1], color="blue", alpha=.1)
plt.scatter(X[i,0], X[i,1], s=150, color="red")
plt.xlim(np.min(X[:,0])-.1, np.max(X[:,0])+.1)
plt.ylim(np.min(X[:,1])-.1, np.max(X[:,1])+.1)
(-0.7736222302611765, 1.344908685522608)
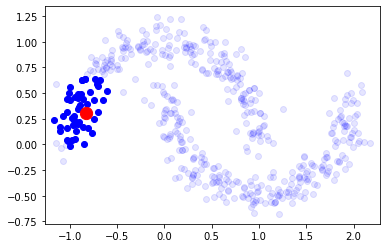
knn_graph
<500x500 sparse matrix of type '<class 'numpy.float64'>'
with 25000 stored elements in Compressed Sparse Row format>
usamos esta matriz de conectividad para suministrar informción de estructura al algoritmo
from sklearn.neighbors import kneighbors_graph
X,_ = make_moons(500, noise=.05)
knn_graph = kneighbors_graph(X, 20, include_self=False)
ac = AgglomerativeClustering(connectivity=knn_graph, linkage="average")
mlutils.plot_cluster_predictions(ac, X, n_clusters=2, cmap=plt.cm.plasma)
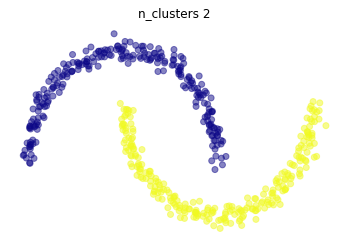
k = knn_graph.todense()
print (k.shape)
k
(500, 500)
matrix([[0., 0., 1., ..., 0., 0., 0.],
[0., 0., 0., ..., 0., 0., 0.],
[1., 0., 0., ..., 0., 0., 0.],
...,
[0., 0., 0., ..., 0., 0., 0.],
[0., 0., 0., ..., 0., 0., 0.],
[0., 0., 0., ..., 0., 0., 0.]])
observa la respuesta a diferentes tamaños de vecindad.
plt.figure(figsize=(15,3))
for i,kn in enumerate([2,20,100]):
plt.subplot(1,3,i+1)
knn_graph = kneighbors_graph(X, kn, include_self=False)
mlutils.plot_cluster_predictions(AgglomerativeClustering(connectivity=knn_graph, linkage="average"), X,
n_clusters=2,
cmap=plt.cm.plasma, show_metric=True, title_str=", kn=%d"%kn)
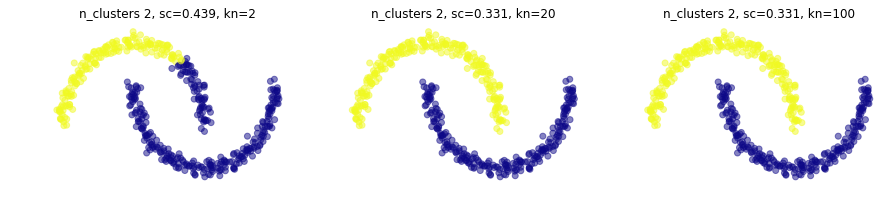
y a distintos niveles de ruido en el dataset
plt.figure(figsize=(15,3))
for i,noise in enumerate([.01,.05,.1]):
plt.subplot(1,3,i+1)
X,_ = make_moons(500, noise=noise)
knn_graph = kneighbors_graph(X, 20, include_self=False)
mlutils.plot_cluster_predictions(AgglomerativeClustering(connectivity=knn_graph, linkage="average"), X,
n_clusters=2,
cmap=plt.cm.plasma, show_metric=True, title_str=", noise=%.2f"%noise)
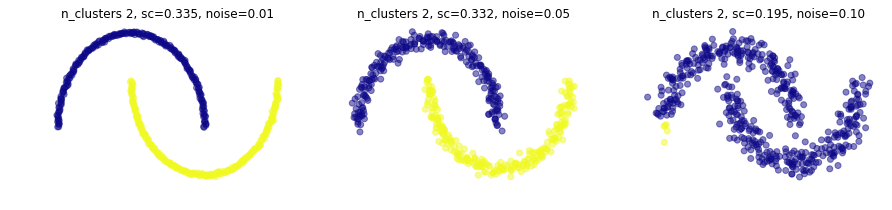
Experimenta¶
observa los resultados de clustering con distintos algoritmos y datasets sintéticos.
Consulta dataset generation en sklearn.
Consulta comparing clustering para datasets sintéticos.
observa en especial si silhouette corresponde a tu intuición.
from sklearn import datasets
X,_ = datasets.make_circles(500, noise=.05, factor=.5)
knn_graph = kneighbors_graph(X, 10, include_self=False)
ac = AgglomerativeClustering(connectivity=knn_graph, linkage="average")
mlutils.plot_cluster_predictions(ac, X, n_clusters=2, cmap=plt.cm.plasma, show_metric=True)
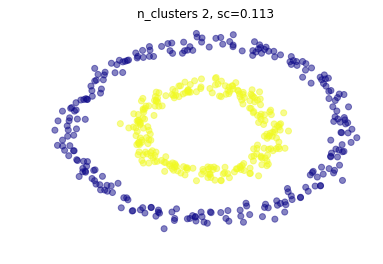
from sklearn import datasets
X,_ = datasets.make_circles(500, noise=.1, factor=.5)
knn_graph = kneighbors_graph(X, 50, include_self=False)
ac = AgglomerativeClustering(connectivity=knn_graph, linkage="average")
mlutils.plot_cluster_predictions(ac, X, n_clusters=2, cmap=plt.cm.plasma, show_metric=True)
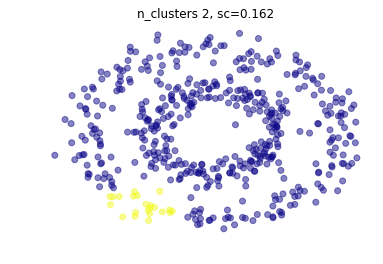
silhouette_analysis(X)
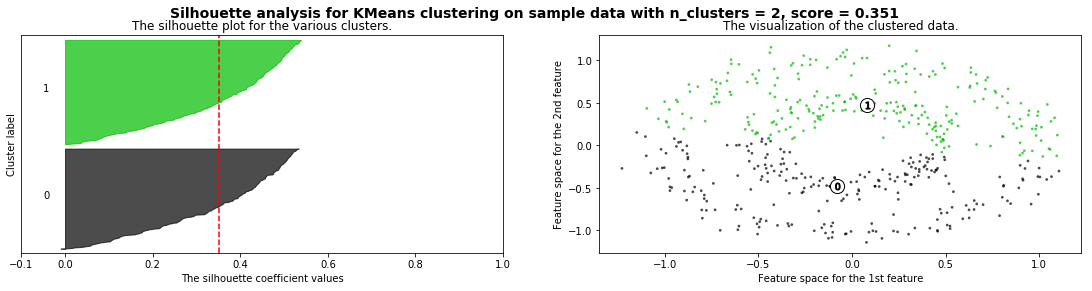
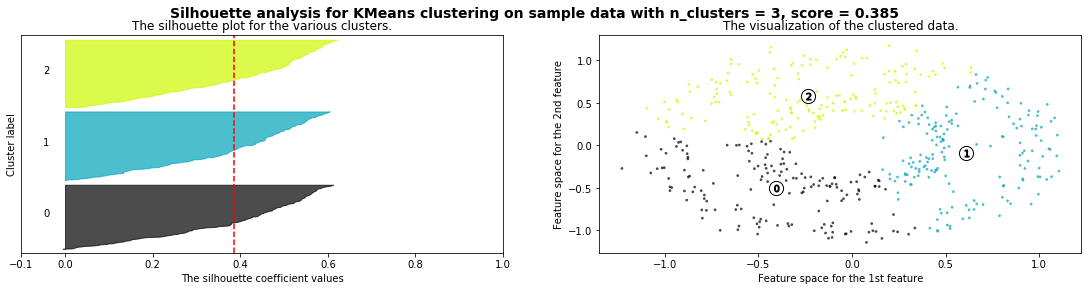
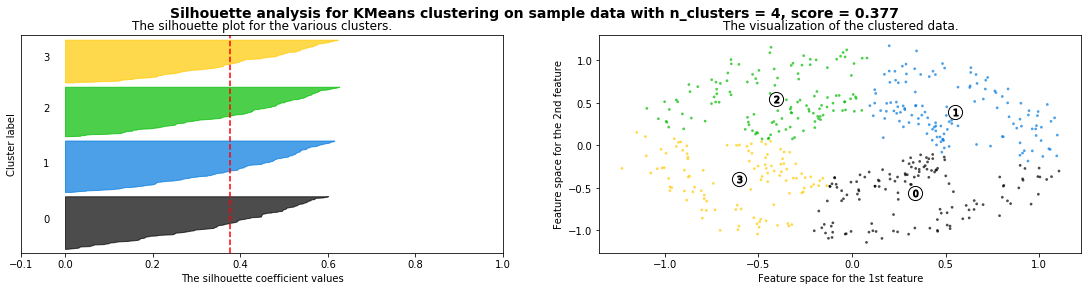
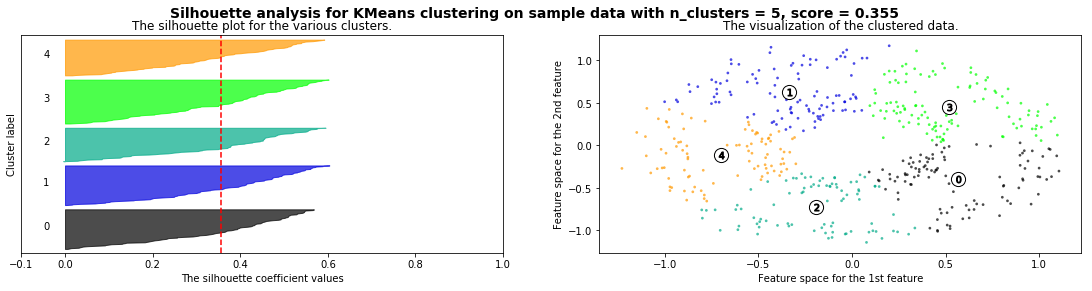
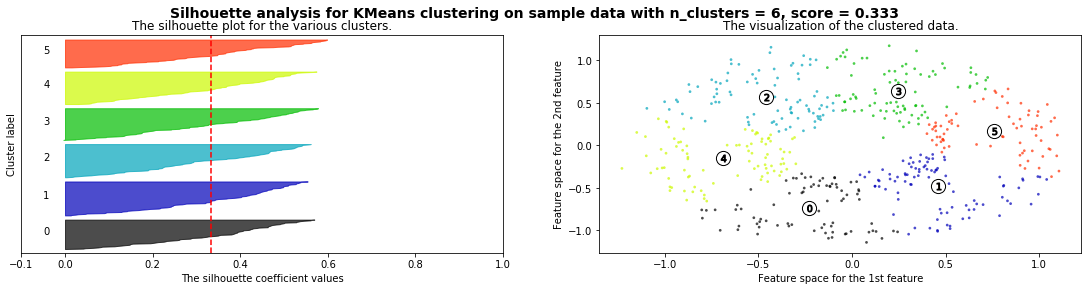