LAB 2.3 - Pairwise classification
Contents
LAB 2.3 - Pairwise classification¶
!wget -nc --no-cache -O init.py -q https://raw.githubusercontent.com/rramosp/2021.deeplearning/main/content/init.py
import init; init.init(force_download=False); init.get_weblink()
from local.lib.rlxmoocapi import submit, session
import inspect
session.LoginSequence(endpoint=init.endpoint, course_id=init.course_id, lab_id="L02.03", varname="student");
LAB SUMMARY¶
In this lab we will use define a slightly different machine learning task. Given two MNIST images as input we want a network with a binary output: 1
if both images belong to the same MNIST class, and 0
otherwise.
import pandas as pd
import numpy as np
import matplotlib.pyplot as plt
from sklearn.model_selection import train_test_split
import tensorflow as tf
%matplotlib inline
mnist = pd.read_csv("local/data/mnist1.5k.csv.gz", compression="gzip", header=None).values
X=(mnist[:,1:785]/255.).astype(np.float32)
y=(mnist[:,0]).astype(int)
print("dimension de las imagenes y las clases", X.shape, y.shape)
Xtr, Xts, ytr, yts = train_test_split(X,y, test_size=0.3)
Xtr.shape, ytr.shape, Xts.shape, yts.shape
FIRST, observe how we build a labelled dataset for this task, so that each training example has:
two images selected randomly from the original dataset
label
0
if they belong to the same class, or1
otherwise
This way, the resulting data structures
pXtr
andpXts
contain the paired images, for train and for testpytr
andpyts
contain the labels of the corresponding paired images, for train and for testeytr
andeyts
contain the0
/1
new labels, one for each pair.
def pairup(X, y, n_pairs_per_class, same_class):
idxs = None
labels = np.unique(y)
for i in labels:
idxs_p1 = np.argwhere(y==i)[:,0] if same_class else np.argwhere(y!=i)[:,0]
idxs_p2 = np.argwhere(y==i)[:,0]
c = np.vstack([
np.random.choice(idxs_p1, size=n_pairs_per_class, replace=True),
np.random.choice(idxs_p2, size=n_pairs_per_class, replace=True)
]).T
idxs = c if idxs is None else np.vstack((idxs,c))
X_pairs = [X[idxs[:,0]],X[idxs[:,1]]]
y_pairs = [y[idxs[:,0]],y[idxs[:,1]]]
y_labels = y[idxs[:,0]]==y[idxs[:,1]]
return idxs, X_pairs, y_pairs, y_labels
def build_image_pairs(X,y,n_pairs_per_class):
idxs0, pX0, py0, ey0 = pairup(X, y, n_pairs_per_class, same_class=False)
idxs1, pX1, py1, ey1 = pairup(X, y, n_pairs_per_class, same_class=True)
pX = [np.vstack((i,j)) for i,j in zip(pX0, pX1)]
py = [np.hstack((i,j)) for i,j in zip(py0, py1)]
ey = np.hstack((ey0, ey1))
idxs = np.vstack((idxs0, idxs1))
return idxs, pX, py, ey.astype(int)
itr, pXtr, pytr, eytr = build_image_pairs(Xtr, ytr, n_pairs_per_class=100)
its, pXts, pyts, eyts = build_image_pairs(Xts, yts, n_pairs_per_class=100)
understand and inspect the structures created and observe how the function above creates a balanced dataset
[i.shape for i in pXtr], [i.shape for i in pytr], itr.shape, eytr.shape
([(2000, 784), (2000, 784)], [(2000,), (2000,)], (2000, 2), (2000,))
[i.shape for i in pXts], [i.shape for i in pyts], its.shape, eyts.shape
([(2000, 784), (2000, 784)], [(2000,), (2000,)], (2000, 2), (2000,))
print ("class distribution %.2f::%.2f"%(np.mean(eytr), 1-np.mean(eytr)))
class distribution 0.50::0.50
for _ in range(5):
i = np.random.randint(len(pXtr[0]))
plt.figure(figsize=(4,2))
plt.subplot(121)
plt.imshow(pXtr[0][i].reshape(28,28), cmap=plt.cm.Greys_r);
plt.ylabel("CLASS %d"%(eytr[i]));
plt.title("pair_0 : %d"%(pytr[0][i])); plt.xticks([],[]); plt.yticks([],[])
plt.subplot(122)
plt.imshow(pXtr[1][i].reshape(28,28), plt.cm.Greys_r);
plt.title("pair_1 : %d"%pytr[1][i]); plt.xticks([],[]); plt.yticks([],[])
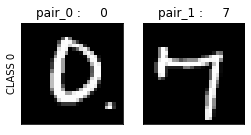
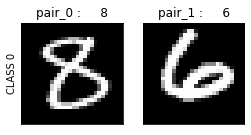
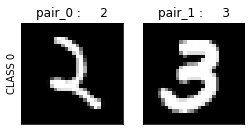
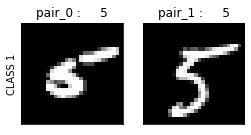
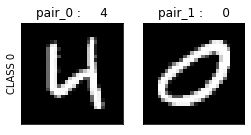
TASK 1: Multi-input model¶
Create a model with the architecture depicted in the following figure.
from IPython.display import Image
Image(filename='local/imgs/twoinputs.png', width=600)
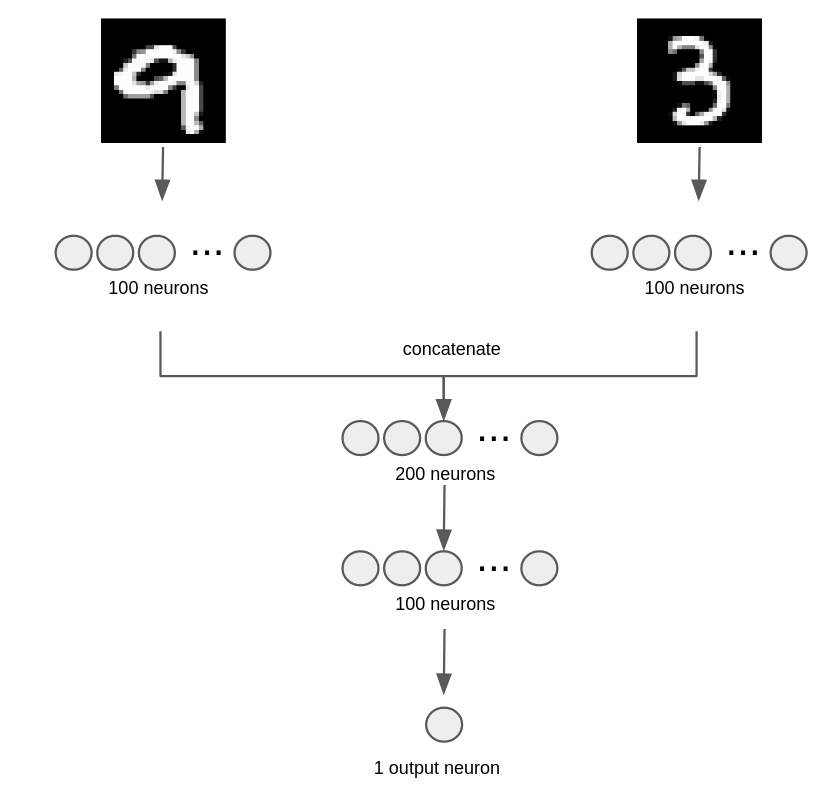
Observe that
it will have TWO input layers with
input_dim=784
neurons each.there is ony ONE output neuron.
the model summary should be as follows and MUST NAME THE LAYERS ACCORDINGLY (but not the
tf.concat
which is just an operation, not a layer):
__________________________________________________________________________________________________ Layer (type) Output Shape Param # Connected to ================================================================================================== input_img0 (InputLayer) [(None, 784)] 0 __________________________________________________________________________________________________ input_img1 (InputLayer) [(None, 784)] 0 __________________________________________________________________________________________________ layer1_img0 (Dense) (None, 100) 78500 input_img0[0][0] __________________________________________________________________________________________________ layer1_img1 (Dense) (None, 100) 78500 input_img1[0][0] __________________________________________________________________________________________________ tf_op_layer_concat_39 (TensorFl [(None, 200)] 0 layer1_img0[0][0] layer1_img1[0][0] __________________________________________________________________________________________________ layer2_common (Dense) (None, 100) 20100 tf_op_layer_concat_39[0][0] __________________________________________________________________________________________________ output (Dense) (None, 1) 101 layer2_common[0][0] ================================================================================================== Total params: 177,201 Trainable params: 177,201 Non-trainable params: 0 __________________________________________________________________________________________________
def get_model(input_dim):
inputs1 = tf.keras.layers.Input(shape=input_dim, name="input_img0")
inputs2 = tf.keras.layers.Input(shape=input_dim, name="input_img1")
...
outputs = ...
model = tf.keras.Model(inputs=[inputs1, inputs2], outputs=outputs)
model.compile(optimizer='adam', loss='mse')
return model
model = get_model(X.shape[1])
model.summary()
Registra tu solución en linea
student.submit_task(namespace=globals(), task_id='T1');
test your model
now we can test your model
model = get_model(X.shape[1])
model.fit(pXtr, eytr, batch_size=16, epochs=20)
preds_ts = (model.predict(pXts)[:,0]>.5).astype(int)
preds_tr = (model.predict(pXtr)[:,0]>.5).astype(int)
print ("accuracy in train data %.2f"%(np.mean(preds_tr==eytr)))
print ("accuracy in test data %.2f"%(np.mean(preds_ts==eyts)))
inspect TEST predictions. Do you see any class getting more confused with others?
for _ in range(20):
i = np.random.randint(len(pXts[0]))
plt.figure(figsize=(4,2))
plt.subplot(121)
plt.imshow(pXts[0][i].reshape(28,28), cmap=plt.cm.Greys_r)
plt.ylabel("PREDICTION %d\nTARGET %d"%(preds_ts[i], eyts[i]))
plt.subplot(122)
plt.imshow(pXts[1][i].reshape(28,28), cmap=plt.cm.Greys_r)
TASK 2: Measure per-class accuracy¶
For each class we want to measure what is the prediction accuracy for the binary task when it participates in a pair. Observe how we gather the labels of each pair together with the binary prediction and the true value.
ts = pd.DataFrame(np.vstack((pyts[0],pyts[1], eyts, preds_ts)).T, columns=["pair_0", "pair_1", "true", "pred"])
ts.head()
pair_0 | pair_1 | true | pred | |
---|---|---|---|---|
0 | 4 | 0 | 0 | 0 |
1 | 8 | 0 | 0 | 0 |
2 | 8 | 0 | 0 | 0 |
3 | 1 | 0 | 0 | 0 |
4 | 2 | 0 | 0 | 0 |
of course, the true
value coincides with pair_0
being equal or different from pair_1
.
np.mean((ts.pair_0==ts.pair_1)==ts.true)
1.0
To compute, per-class accuracy in this task, for instance for class 2:
select the rows where
pair_0
orpair_1
is 2measure the percentage of time in the selected rows where
true==pred
for instance, for the following DataFrame
pair_0 pair_1 true pred
0 0 0 1 1
1 0 0 1 1
2 0 0 1 1
3 2 2 1 1
4 1 1 1 1
5 0 2 0 0
6 2 2 1 0
7 2 2 1 1
8 2 2 1 1
9 1 1 1 1
10 1 1 1 1
11 1 1 1 1
12 2 2 1 1
13 0 2 0 1
14 2 2 1 1
15 0 0 1 1
16 2 2 1 1
17 1 1 1 0
18 1 1 1 1
19 1 1 1 1
you must return this accuracies:
{0: 0.8333333333333334, 1: 0.8571428571428571, 2: 0.7777777777777778}
the accuracies must be returned as a dictionary such as above. They keys are the original class labels, and the values the accuracy just described.
The accuracies must be correct up to 3 decimal values.
def perclass_bin_accuracy(ts):
r = {}
for i in np.unique(ts.pair_0.tolist() + ts.pair_1.tolist()):
...
r[i] = ... # your code here
return r
test your code with the example above
t = pd.DataFrame(
np.array([[0, 0, 1, 1],[0, 0, 1, 1],[0, 0, 1, 1],[2, 2, 1, 1],[1, 1, 1, 1],
[0, 2, 0, 0],[2, 2, 1, 0],[2, 2, 1, 1],[2, 2, 1, 1],[1, 1, 1, 1],[1, 1, 1, 1],[1, 1, 1, 1],
[2, 2, 1, 1],[0, 2, 0, 1],[2, 2, 1, 1],[0, 0, 1, 1],[2, 2, 1, 1],[1, 1, 1, 0],[1, 1, 1, 1],
[1, 1, 1, 1]]),
columns=["pair_0", "pair_1", "true", "pred"])
perclass_bin_accuracy(t)
test your code with other random examples
n, n_classes = 20, 3
p0 = np.random.randint(n_classes, size=n)
p1 = np.random.randint(n_classes, size=n)
dtrue = (p0==p1).astype(int)
preds = np.random.randint(2, size=n)
td = pd.DataFrame([p0,p1,dtrue,preds], index=["pair_0", "pair_1", "true", "pred"]).T
td
perclass_bin_accuracy(td)
Registra tu solución en linea
student.submit_task(namespace=globals(), task_id='T2');